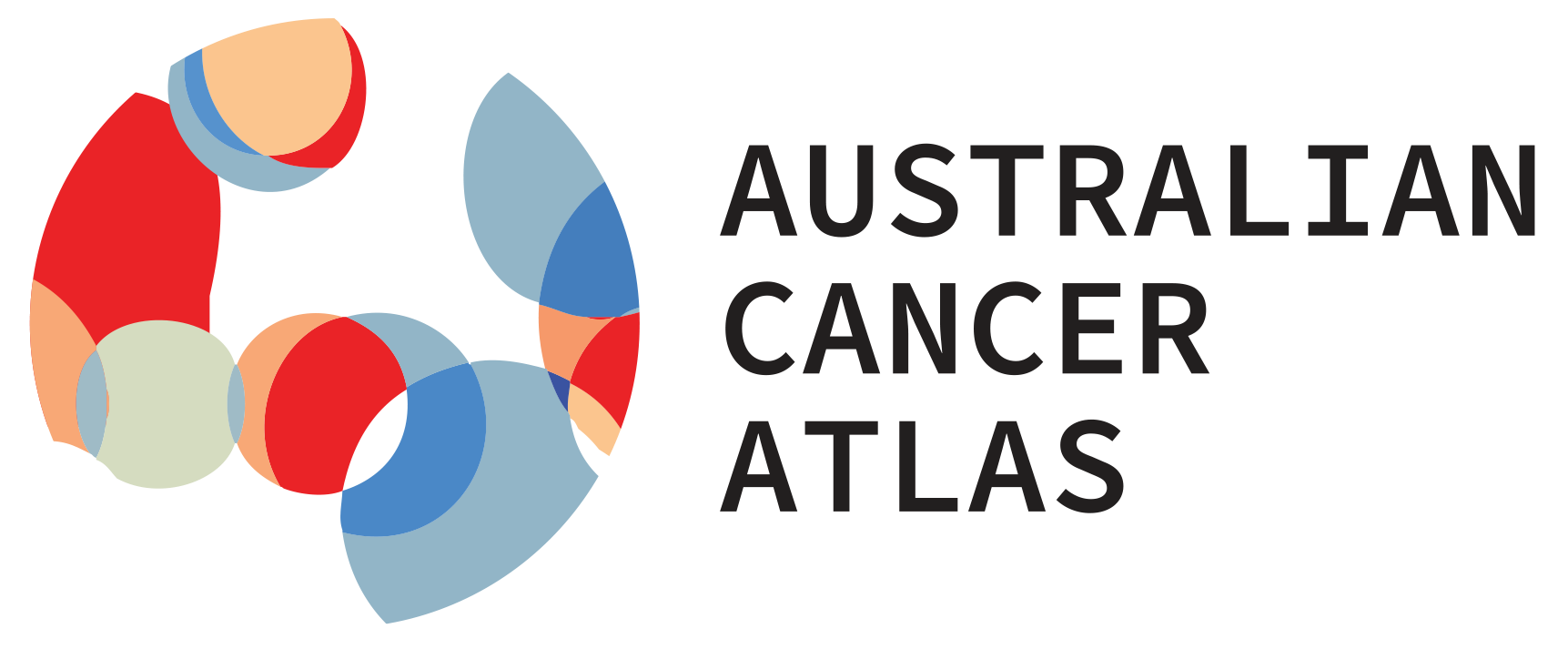
9 Cancer survival
9.1 What is cancer survival?
The term “cancer survival” refers to the mortality among people diagnosed with cancer. While it is possible to calculate mortality due to any cause, the main interest is typically on the mortality associated with the diagnosis of cancer; so, whether a person with colorectal cancer dies of colorectal cancer, rather than dying from another cause such as a car accident.
Determining the exact cause of death can be difficult, particularly when someone has several medical conditions at the same time, for example dementia and breast cancer. This can make it difficult to calculate what is known as “cause-specific survival”, because it is not known what the single cause of death is.
One way of addressing this is to use “relative survival” where the all-cause survival for the group of cancer patients is compared with the all-cause survival of the general population. This comparison is matched by age group, so that, any difference in survival between the two groups can be attributed to the diagnosis of cancer.
A 5-year relative survival estimate of 100% indicates that, on average, there is no difference in the survival experience between the group of cancer patients and the general population over the period within five years of diagnosis.
A 5-year relative survival estimate of 50% indicates that, on average, the group of cancer patients is about half as likely to survive for five years than would be expected in the general population.
9.2 Cancer types
The Australian Cancer Atlas reports geographical patterns in survival for most cancer types included in the diagnosis estimates (See Section 8.2). Some cancer types were excluded from the survival modelling because they have very high survival rates (>97%). The cancer types excluded from survival modelling were testicular cancer, in situ cancers (melanoma, female breast), thin melanomas (that is, invasive melanomas with a thickness less than 1 mm) and localised cancers (melanoma, female breast, prostate). Many of these cancer types are subsets of other cancer types, however, the survival models for invasive breast cancer, invasive melanoma and invasive prostate cancer included both localised and advanced cancer diagnoses and both thick and thin melanomas. In addition, thyroid cancer was also excluded from the survival modelling due to concerns about the stability of the modelled estimates.
9.3 Data sources
9.3.1 Cancer registry
Cancer survival outcomes were calculated using the cancer registry data from the Australian Cancer Database. (Australian Cancer Database) Further details can be found in Section 8.3.1.
Cancer registries routinely link with the National Death Index (National Death Index) to determine whether people who have been diagnosed with cancer have died. For people who were identified as having died, this linkage provides details about the time between diagnosis and death.
The Australian Cancer Atlas reports survival estimates for Australians aged 15 to 89 years. Patients whose cancer diagnosis was based on death certificate or autopsy only were also excluded, as well as those with a survival time of zero days or a date of diagnosis after date of death.
9.3.2 Population
Population data by SA2, five-year age group and year was obtained from the Australian Bureau of Statistics. (Australian Bureau of Statistics, 2022a)
9.3.3 Population mortality data
Population mortality data for Australia by sex, year of death and single-year age group (15,16…100+ years) was obtained from the Australian Bureau of Statistics. (Australian Bureau of Statistics, 2022b). Please see Section 6.1 for details.
Population mortality data by small areas were obtained by smoothing mortality rates by single-year age, year and SA2 (see Section 6.2) based on unit record file mortality data obtained either from the Australian Bureau of Statistics (2001 to 2005) or from the Registries of Births, Deaths and Marriages from 2006 to 2020. (Registry of Births Deaths and Marriages, 2023)
9.3.4 Geographical areas
The data set included details on the usual area of residence at time of cancer diagnosis, based on their 2016 SA2. (Australian Bureau of Statistics, 2016a) For a small subset of records, the known 2011 SA2 information was converted to 2016 SA2 using population-weighted geographical correspondence files. (Australian Bureau of Statistics Geospatial Solutions, 2023) Records with no SA2 information were excluded.
Of 2,288 SA2 (2016) areas in Australia that were potentially eligible for analysis after excluding 18 non-spatial SA2s and four remote islands (see Section 4.1), another 50 SA2s that had an annual average resident population of fewer than five residents during 2010-2019 were also excluded. This means the Australian Cancer Atlas provides modelled estimates for cancer survival for 2,238 SA2s across Australia.
9.3.5 Clinical characteristics
The Australian cancer atlas reports geographical patterns in survival by cancer stage at diagnosis for breast, prostate and melanoma, and melanoma thickness at diagnosis across Australia. Estimates were calculated using the cancer diagnosis data by clinical characteristics. (see Section 8.3.4) These clinical characteristics are important determinants of survival from the respective cancers.
Due to very high survival for in situ cancers and thin melanomas, survival estimates are not reported for these combinations.
9.4 Statistical methods
9.4.1 National summary measures
Number of people surviving 5 years after a cancer diagnosis
The number of people per year surviving at least five years provides an estimate of the people who were living five years after being diagnosed with the selected cancer type.
Survival estimates were calculated using relative survival methods. Relative survival is the most commonly presented measure of cancer survival when using data from population-based cancer registries. (Dickman et al., 2004) One key advantage is that no knowledge of the specific cause of death is needed.
Relative survival estimates can be calculated using either the period or cohort methods, (Brenner et al., 2004) and we used the period approach. Rather than following a cohort diagnosed within a certain time period, this examines anyone alive within 5-years of diagnosis within a certain time period.
The life table method was used to calculate observed survival. This approach involves dividing the total period being studied into a series of discrete time intervals. Survival probabilities were calculated for each of these intervals, and then multiplied together to produce the observed survival estimate. Expected survival based on total Australian mortality data (see Section 6.1) was calculated for each age (15,16,17,…,100+ years) and individual calendar year (2010, 2011,…,2019) based on the Ederer II method. (Ederer et al., 1961)
Any follow-up time intervals (that is time since diagnosis) extending past five years were excluded, and the number of people alive at the start of each follow-up interval minus the number of observed deaths was summed and divided by ‘at-risk’ time period (for example 10 if time period is 2010-2019) to provide an average per year.
Relative survival rate
The 5-year survival rate was calculated as percent relative survival. Here relative survival was calculated as above, but also internally age standardised. This involved calculating the proportion of cases diagnosed among broad age groups (15-54, 55-64, 65-74, 75-89 years) and weighting the relative survival estimates for each of these age groups by this proportion.
9.4.2 Interpretation of reported geographical estimates
When diagnosed with a cancer, a person may ask “How likely am I to be alive in five years?” or conversely “How likely am to die from this?”.
There are many ways to answer this question. The statistical models used for the Australian Cancer Atlas are based on the numbers of deaths within five years of diagnosis for people diagnosed with cancer, and comparing these to the expected number of deaths if that group had the same mortality rates as the general population. This ratio is known as relative survival.
To compare the survival of people across Australia, the relative survival for a specific area is compared to the Australian average over the same period.
In the Australian Cancer Atlas, areas shown in orange or red have worse cancer survival within five years of diagnosis than the Australian average, while areas shown in blue have better cancer survival within five years of diagnosis than the Australian average.
The survival estimates in the Australian Cancer Atlas reflect the relative survival from a cancer within a specific geographic area for people “at-risk”, that is, people within five years of a cancer diagnosis. All the estimates presented in the Australian Cancer Atlas are about the average cancer burden for all people living within a specific geographical area. Since people are different, with different lifestyles and habits, this overall survival will not reflect the survival for every individual living in that area.
9.4.3 Spatial model for survival (excess deaths)
The spatial model for survival was a Poisson Bayesian spatial relative survival model, closely based on that first proposed by Fairley and colleagues (Fairley et al., 2008) but using the Leroux prior. (Cameron et al., 2022, Dasgupta et al., 2023a, Duncan et al., 2019a) The first stage is the likelihood model for the observed number of deaths for age group \(k\) and area \(i\) and follow-up interval \(j\), \(d_{ijk}\ \)which was Poisson with mean \(\mu_{ijk}\):
\[d_{ijk}\ \sim\ Poisson(\mu_{ijk})\]
The second stage models the excess number of deaths (\(\mu_{ijk} - d_{ijk}^{*}\)), offset by the persons at risk (\(y_{ijk}\)) with adjustments for age, sex and cancer sub-type. A separate intercept, \(\beta_{j}\), was fitted for each “follow-up year” \(j\), which is the number of years since diagnosis:
\[\log\left( \mu_{ijk} - d_{ijk}^{*} \right) = \log\left( y_{ijk} \right) + \alpha_{j} + x\beta_{k} + S_{i}\]
where \(d_{ijk}^{*}\) is the expected number of deaths according to the area- and age-specific population mortality, \(y_{ijk}\) is the person-time at risk, \(\alpha_{j}\) is the intercept (which varied by follow-up year), \(\beta_k\) is the coefficient of the predictor variable vector \(x\) (representing the broad age groups, sex and cancer type). The spatial random effect \(\text{S}_{i}\) were modelled with the Leroux prior, (Leroux et al., 2000) which is a Gaussian distribution, and its mean is the weighted average of the neighbouring areas’ random effects (with weight \(\rho\)) and a global mean of 0 (with weight \(1 - \ \rho\)).
\[S_{i}|S_{\backslash i}\ \sim\ N\left( \frac{\rho\Sigma_{h}w_{ih}S_{h}}{\rho\Sigma_{h}w_{ih} + 1 - \rho},\ \frac{\sigma_{S}^{2}}{\rho\Sigma_{h}w_{ih} + 1 - \rho} \right)\]
The spatial weights \(w_{ih}\) represent the spatial proximity between areas \(i\) and \(h\), and collectively they comprise an N×N spatial adjacency matrix W. A binary weighting scheme was used, so that \(w_{ih} = 1\) if areas \(i\) and \(h\) were considered to be neighbours, and otherwise \(w_{ih} = 0\).
The variance \(\sigma_{S}^{2}\) was given a weakly informative prior, where \(HN\) refers to the half-normal distribution. The priors for the intercept, regression coefficients, and spatial smoothing parameter \(\rho\) were weakly informative.
\[\beta_{j}\ \sim\ N(0,0.01)\]
\[\beta_{k}\ \sim\ N(0,0.01)\]
\[\sigma_{S}^{2}\ \sim\ HN(1,\ 0.2)\]
\[\rho\ \sim\ Uniform(0,1)\]
The key output used in the maps from this model is the survival ratio (SR), which was calculated as the posterior median of \(1/exp\left( S_{i} \right)\).
The model was run separately for males, females, and persons. The covariates included were to prevent bias in the estimates due to differences between areas in the age structures (4 age groups, representing ages 15-54, 55-64, 65-74 and 75-89 years), sex (only for persons, separated into males and females) and cancer types (only for cancer types that grouped together cancer sub-types with different survival outcomes). This included the models for all cancers, rare cancers, rare blood cancers, oral cancers, head and neck cancers, neuroendocrine cancers, soft tissue sarcomas, and classic myeloproliferative neoplasms.
The period approach and life table method were used to calculate the observed number of deaths, the person-time at risk and the age- and sex-matched population mortality. This approach involves dividing the time after diagnosis into a series of discrete time intervals. Five annual intervals were used, and comparisons against models using quarterly intervals for the first year showed no differences in the estimates. Person-time at risk was also obtained from this, as it reflects the sum of the patients alive within each discrete time interval.
9.4.3.1 Bayesian spatial models for cancer survival
9.4.3.1.1 Input data set
Each row of the input data set represented a unique small area, age group, risk interval, sex and cancer group. “Risk interval” refers to the number of years since diagnosis. The cancer group variable is for cancer types with multiple subtypes with differing survival outcomes.
Variable | Value |
---|---|
idarea | A unique identification number for each small area. The numbers should be consecutive values from 1 to N, where N is the total number of areas |
RiskInterval | Coded as 1-5, where 1 is the first year after diagnosis and 5 is the fifth |
bagegrp | There were 4 age groups representing ages 15-54, 55-64, 65-74 and 75-89 years |
d | Observed number of deaths by area, age group, sex, and risk interval (from strs) |
d_star | The population mortality for the area, reflecting the age and sex of the persons at risk (from strs) |
y | The person-time at risk |
sex | For modelling all persons only. Coded 1 for males and 2 for females |
cancergrp | For modelling types of cancer with subtypes that have differing survival outcomes |
The data were prepared using the strs command in Stata.
Dickman, PW; Coviello, E. 2015. Estimating and modeling relative survival, Stata J, 15(1):186-215.
9.4.3.1.2 Adjacency matrix
An adjacency matrix specifies the spatial structure of the small areas, with a unique row and column for each area. For the Australian Cancer Atlas, a first-order adjacency matrix was used, in which two areas were considered neighbours if they shared a common geographical boundary. Small areas without any shared boundaries (for example islands) were manually assigned at least one neighbour (usually the closest mainland area).
The column and row number for each area corresponds to the area’s unique identification number (“idarea”, as described above). The \((i,{h)}^{th}\) element of the matrix was 1 if areas \(i\) and \(h\) share a border, and 0 if \(i = h\) or if areas \(i\) and \(h\) do not share a border. The matrix must be symmetric, that is the \((i,{h)}^{th}\) element is equal to the \(({h,i)}^{th}\)element.
9.4.3.1.3 Stata code
// Final year of follow-up
global current = 2019
// Run stset
stset exit, enter(time mdy(1,1,`=$current-9')) exit(time mdy(12,31,$current))\\\
origin(datediag) failure(death=1) id(id) scale(365.24)
// Calculate population mortality, observed deaths and person-time at risk by area,
// age group, sex and cancer group
// using filename specifies a file containing general-population survival
// probabilities (conditional probabilities of surviving one year), stratified by area,
// age, sex, and calendar year. Age must be specified in one-year increments
(up to maxage specified) and calendar year in one-year intervals.
// The data must be sorted by the variables specified in mergeby().
// Default names for variables in this file are prob for the survival
// probabilities, _age for age and _year for calendar year.
strs using popmort_area_9619, br(0(1)5) mergeby(idarea _year sex _age) \\\
by(sex bagegrp cancergrp year idarea) diagage(age) diagyear(year) maxage(89) \\\
savgr(grouped.dta, replace) savind(individ.dta, replace)
use individ.dta, clear
bys id start: gen check = _n
keep if check == 1
rename start RiskInterval
// Aggregate by area, risk interval, age group, sex and cancer group
sort idarea RiskInterval bagegrp sex cancergrp
collapse (sum) y d_star d, by(idarea RiskInterval bagegrp sex cancergrp)
save input_data.dta, replace
9.4.3.1.4 R code
library(R2WinBUGS)
# W is the adjacency matrix
# The input data, 'dat.in' are as described in the above table, where
# idarea is the unique area ID and corresponds to the area's row / column number in W
# RiskInterval is the number of years since diagnosis
# bagegrp is the age group (1 is 15-54, 2 is 55-64, 3 is 65-74 and 4 is 75-89 years)
# sex is 1 for males and 2 for females
# cancergrp is for types of cancers with multiple sub-types that have varying survival outcomes
# d is the observed number of deaths
# d_star is the mortality in the general population corresponding to the age, sex and area of the persons at risk
# y is the persons-time at risk
# Obtain adjacency information
adj <- W2adj(W)
# Set constants
N <- length(adj$num) # Number of areas
RiskYear <- data.in$RiskInterval + 1
nRiskYears = length(unique(dat.in$RiskInterval))
nage = length(unique(dat.in$bagegrp))
ngroup = length(unique(dat.in$cancergrp))
nsex = length(unique(dat.in$sex))
nage = nage - 1
ngroup = ngroup - 1
nsex = nsex - 1
## Create data list to be passed to WinBUGS
bugs.dat<- list(n = nrow(dat.in),
d = dat.in$d,
d.star = dat.in$d_star, y = dat.in$y,
RiskYear = RiskYear,
nareas = N,
cum = c(cumsum(adj$num)-adj$num, sum(adj$num)),
sumnum = sum(adj$num), adj = adj$adj, num = adj$num,
agegp1 = as.numeric(dat.in$bagegrp == 1),
agegp2 = as.numeric(dat.in$bagegrp == 2),
agegp4 = as.numeric(dat.in$bagegrp == 4),
sex = as.numeric(dat.in$sex == 2),
cancergrp = as.numeric(dat.in$cancergrp == 2),
areaid = dat.in$idarea,
nRiskYears = nRiskYears
)
# get the total number of covariates
num.covariates = nage + nsex + ngroup
# Initial values
inits <- function(){
list(
alpha = rep(-3, 5),
u = rep(0, nareas),
sigma.u2 = 1/5,
rho = 0.5,
beta = rep(0, num.covariates)
)
}
# Set parameters to monitor in WinBUGS
parameters <- c("alpha", "u", "sigma.u2", "beta", "rho")
# source(model.code)
bugs.file <- model.code
## Run WinBUGS model code
MCMC.bugs <- bugs(
data = bugs.dat,
inits = inits,
parameters.to.save = parameters,
model.file = bugs.file,
n.chains = 1,
n.iter = 150000,
n.burnin = 5000,
n.thin = 10,
debug = FALSE,
bugs.directory = "C:/WinBUGS14/",
program = c("WinBUGS"),
DIC = FALSE
)
9.4.3.1.5 WinBUGS code
model.code <- WinBUGSCode({
for (idx in 1:n) {
d[i] ~ dpois(mu[i])
mu[i] <- d.star[i] + d.excess[i]
log(d.excess[i]) <- log(y[i]) + alpha[RiskYear[i]] + beta[1] * agegp1[i] +
beta[2] * agegp2[i] + beta[3] * agegp4[i] + beta[4] * sex[i] +
beta[5] * cancergrp [i] + u[Area[i]]
}
# Leroux prior for spatial effects
for(j in 1: nareas){
u[j] ~ dnorm(mean.u[j], prec.u[j])
A[j] <- (rho * num[j] + 1 - rho)
prec.u[j] <- A[j] / sigma.u2
mean.u[j] <- rho * sum(W.u[cum[j] + 1:cum[j+1]]) / A[j]
}
for (h in 1:sumnum) {
W.u[h] <- u[adj[h]]
}
# Other priors
sigma.u2 ~ dnorm(0, 0.2)I(0,)
rho ~ dunif(0, 1)
for(t in 1: nRiskYears){ #flat prior on intercept
alpha[t] ~ dnorm(0, 0.01)
}
for(k in 1:5){
beta[k] ~ dnorm(0, 0.01)
}
})
9.4.4 Modelled number of cancer survivors at 5-years
The Australian Cancer Atlas also includes an absolute measure of cancer survival, which is the modelled number of people in a specific geographical area who have survived at least five years since their cancer diagnosis.
These calculations used the modelled number of cancer diagnoses from the fitted spatial diagnosis models, the results of the spatial survival models and the population mortality to estimate the modelled number of deaths for each geographical area after five years. The number of people living five years after the diagnosis was then calculated by subtracting the modelled number of deaths from the modelled number of diagnoses.
9.4.5 Spatiotemporal models
The spatiotemporal survival model was based on the spatial model for survival first described by Fairley and colleagues, (Fairley et al., 2008) the spatiotemporal models of Bernardinelli and colleagues (Bernardinelli et al., 1995) and the implementation of splines by Crainiceanu and colleagues. (Crainiceanu et al., 2005)
In the first stage, the number of deaths observed in area \(i\), age group \(k\), in the \(j\)th year since diagnosis and at time point \(t\) (\(d_{ijkt}\)) was modelled as a Poisson process:
\[d_{ijkt}\ \sim\ Poisson\left( \mu_{ijkt} + d_{ijkt}^{*} \right)\ \ \ \ for\ i = 1,\ldots,\ 2238\ areas\ and\ t = 1,\ldots,T\ time\ points\]
where \(\mu_{ijkt}\) was the modelled number of excess deaths and \(d_{ijkt}^{*}\) was the number of deaths that might be expected to have occurred in the cancer cohort if they had not been diagnosed with cancer. This number was based on the population mortality statistics for each area, age group and sex.
The second stage models the excess deaths (\(\mu_{ijkt}\)):
\[\log\left( \mu_{ijkt} \right) = \log\left( y_{ijkt} \right) + \alpha_{j} + \beta_{k} + \zeta_{s} + \ f(t) + f_{i}(t) + R_{i}\]
where \(y_{ijkt}\) is the person-time at risk, \(\alpha_{j}\) is the intercept for the \(j\)th year since diagnosis, \(\beta_{k}\) is the effect for age group \(k\), \(\zeta_{s}\) is the effect of sex, \(f(t)\) is the national temporal trend, \(R_{i}\) is the spatial effect and \(f_{i}(t)\) is the area-specific deviations from the national trend.
The key output from these models is the survival ratio (\(SR_{i}\)), which was calculated as the inverse of the excess hazard ratio (\(EHR_{i})\). If the type of estimate was “Changes over time for each geographical area”, the calculation of the SR included the national temporal trend.
\[EHR_{i} = \frac{1}{SR_{i}} = \exp\left( f(t) + f_{i}(t) + R_{i} \right)\]
If the type of estimate was “Geographical patterns (separate time periods)”, the calculation of the SR included only the spatial effect and the area deviation from the national trend.
\[EHR_{i} = \frac{1}{SR_{i}} = \exp\left( f_{i}(t) + R_{i} \right)\]
Spatial effects
The spatial effects were modelled by a BYM2 prior, (Riebler et al., 2016) which is a weighted average between a spatially structured effect (\(\phi_{i}\)) and a Gaussian effect with zero mean (\(\theta_{i}\)):
\[\theta_{i}\ \sim\ N(0,\ 1)\]
\[\phi_{i}|\phi_{\backslash i}\ \sim\ N\left( \frac{\sum_{h}^{}{w_{ih}\phi_{h}}}{\sum_{h}^{}w_{ih}},\ \frac{1}{\sum_{h}^{}w_{ih}} \right)\]
\[R_{i} = \frac{1}{\sqrt{\tau_{R}}}\left( \theta_{i}\sqrt{1 - \rho} + \ \phi_{i}\sqrt{\rho/\iota} \right)\]
where \(\iota\) is a scaling factor calculated from the adjacency matrix, W, which is comprised of the spatial weights \(w_{ih}\). These weights represent the spatial proximity between areas \(i\) and \(h\). A binary weighting scheme was used, so that \(w_{ih} = 1\) if areas \(i\) and \(h\) were considered neighbours and \(w_{ih} = 0\) otherwise.
Temporal effects
The national trend and the area deviations from the national trend were modelled using thin-plate splines. (Crainiceanu et al., 2005) Splines are a set of simple lines or curves added together, resulting in a versatile range of possible curves. You can explore splines in the app below.
The national temporal trend was modelled by a linear component (\(\gamma\)) and a set of spline coefficients (\(g_{\kappa}\) for each knot \(\kappa = 1,\ldots,Κ\)), as follows:
\[f(t) = \gamma X_{t} + \sum_{\kappa = 1}^{Κ}{g_{\kappa}Z_{\kappa,t}}\]
where \(X_{t}\) is the time point (normalised) and \(Z_{\kappa,\ t}\) is the spline for the \(\kappa\)th knot at time point \(t\).
For some cancer types, the areas’ deviations from the national trend were modelled as a line and others were modelled as a thin plate spline with two knots.
\[f_{i}(t) = \left\{ \ \begin{array}{r} h_{i}X_{t} \\ \sum_{\kappa = 1}^{2}{h_{i,\kappa}{Z_{areas}}_{\kappa,t}} \\ \end{array} \right.\ \ \]
For some cancer types, the temporal trend was linear and in this case the area deviation was also linear.
For each combination of cancer type and sex, multiple models were fitted with different numbers of knots. Initially, multiple numbers of knots were fitted to the national data using a temporal model (as above, but without \(R_{i}\) or \(f_{i}(t)\)) and the optimal number of knots, \(Κ_{opt}\), for each cancer type and sex was identified using the WAIC. (Watanabe, 2010) A set of candidate numbers of knots for each cancer type and sex was then defined for the spatiotemporal models as \(\left\{ 1,\ 2,\ \ Κ_{opt} - 1,\ Κ_{opt} \right\}\). In practice, \(Κ_{opt}\) was typically one or two and so only one or two knots were fitted to most cancer types. After the spatiotemporal models were fitted using this set of candidates, the best number of knots fitted to each type of cancer and sex was selected using WAIC, Moran’s ST of the residuals (Anderson and Ryan, 2017) and other model fit considerations.
Other priors and hyperpriors
The intercepts and coefficients were given Gaussian priors, each with unique precision. For example, the priors on the intercepts for the risk intervals were as shown below.
\[\beta_{j}\ \sim\ N(0,\ \tau_{\beta})\]
\[\tau_{\beta}\ \sim\ Gamma(1,\ 0.01)\]
Similarly, the precision on the spatial random effects also had a gamma prior.
\[\tau_{R}\ \sim\ Gamma(1,\ 0.01)\]
The mixing parameter was given a beta prior with uniform distribution.
\[\rho\ \sim\ beta(1,\ 1)\]
9.4.5.1 Bayesian spatiotemporal models for cancer survival
9.4.5.1.1 Input data set
Each row of the input data set represented a unique time point, small area, age group, risk interval, sex and cancer group. “Risk interval” refers to the number of years since diagnosis. The cancer group variable is for cancer types with multiple subtypes with differing survival outcomes.
Variable | Value |
---|---|
idarea | A unique identification number for each small area. The numbers should be consecutive values from 1 to N, where N is the total number of areas |
yeargrp | The time point (year or year group) |
RiskInterval | Coded as 1-5, where 1 is the first year after diagnosis and 5 is the fifth |
bagegrp | There were 4 age groups representing ages 15-54, 55-64, 65-74 and 75-89 years |
d | Observed number of deaths by area, time, age group, sex, and risk interval (from strs) |
d_star | The population mortality for the area and time point, reflecting the age and sex of the persons at risk (from strs) |
y | The person-time at risk |
sex | For modelling all persons only. Coded 0 for males and 1 for females |
cancergrp | For modelling types of cancer with subtypes that have differing survival outcomes |
The data were prepared using the strs command in Stata.
Dickman, PW; Coviello, E. 2015. Estimating and modeling relative survival, Stata J, 15(1):186-215.
9.4.5.1.2 Adjacency matrix
An adjacency matrix specifies the spatial structure of the small areas, with a unique row and column for each area. For the Australian Cancer Atlas, a first-order adjacency matrix was used, in which two areas were considered neighbours if they shared a common geographical boundary. Small areas without any shared boundaries (for example islands) were manually assigned at least one neighbour (usually the closest mainland area).
The column and row number for each area corresponds to the area’s unique identification number (“idarea”, as described above). The \((i,{h)}^{th}\) element of the matrix was 1 if areas \(i\) and \(h\) share a border, and 0 if \(i = h\) or if areas \(i\) and \(h\) do not share a border. The matrix must be symmetric, that is the \((i,{h)}^{th}\) element is equal to the \(({h,i)}^{th}\)element.
9.4.5.1.3 Stata code
// Final year of follow-up
global current = 2019
// First year that has 5-years of follow-up data
global entry = 2001
// Width of year groups (our yeargrp included two calendar years)
global width = 2
// Run stset
stset exit, enter(time mdy(1,1,$entry)) exit(time mdy(31,12,$current)) \\\
origin(datediag) failure(death=1) id(id) scale(365.24)
// Calculate population mortality, observed deaths and person-time at risk by \\\
area, year, age group, sex and cancer group
strs using popmort_area_9619, br(0(1)5) mergeby(idarea _year sex _age) \\\
by(sex bagegrp cancergrp year idarea) diagage(age) diagyear(year) maxage(89) \\\
savgr(grouped.dta, replace) savind(individ.dta, replace)
use individ.dta, clear
bys id start: gen check = _n
keep if check == 1
rename start RiskInterval
// How many time points will there be in the data set
local J = floor(($current -- $entry + 1) / $width)
// What is the first year of data to be included
local y0 = $current - `J' * $width + 1
// Construct a variable for the time point, "yeargrp"
gen yeargrp = floor((_year --`y0' - $width) / $width + 1) + 1
keep if yeargrp >= 1
// Construct a label for "yeargrp"
local inc = 0
forvalues tt = `y0'($width)\$current {
local inc = `inc' + 1
la def zyeargrp `inc' "`tt'-`=`tt' + $width - 1'", modify
}
la val yeargrp zyeargrp
// Aggregate by area, year group, risk interval, age group, sex and cancer group
sort idarea yeargrp RiskInterval bagegrp sex cancergrp
collapse (sum) y d_star d, by(idarea yeargrp RiskInterval bagegrp sex cancergrp)
save input_data.dta, replace
9.4.5.1.4 R code
library(nimble)
# W is the adjacency matrix
# The input data, 'dat.in' are as described in the above table, where
# idarea is the unique area ID and corresponds to the area's row / column number in W
# yeargrp is the time point
# RiskInterval is the number of years since diagnosis
# bagegrp is the age group (1 is 15-54, 2 is 55-64, 3 is 65-74 and 4 is 75-89 years)
# sex is 0 for males and 1 for females
# cancergrp is for types of cancers with multiple sub-types that have varying survival outcomes
# d is the observed number of deaths
# d_star is the mortality in the general population corresponding to the age, sex and area of the persons at risk
# y is the persons-time at risk
# num.knots is the number of knots to be fitted. This code assumes that num.knots > 1
# The number of areas (from the adjacency matrix)
N = ncol(W)
# Obtain scaling factor for BYM2 from adjacency matrix
# ICAR precision matrix
Q = apply(W, 1, sum) * diag(N) - W
# Add a small jitter for numerical stability
Q_pert <- Q + diag(N) * max(diag(Q)) * sqrt(.Machine$double.eps)
# Compute the diagonal elements of the covariance matrix subject to the
# constraint that the entries of the ICAR sum to zero
Q_inv <- INLA::inla.qinv(Q_pert, constr = list(A = matrix(1,1,N), e=0))
# Compute the geometric mean of the variances (diagonal of Q_inv)
scaling.factor <- exp(mean(log(diag(Q_inv))))
# Obtain adjacency information
adj.info <- as.carAdjacency(W)
L = length(adj.info$adj)
# Get standardised time variable
time.ids <- sort(unique(dat.in$yeargrp))
dat.in$idtime <- match(dat.in$yeargrp, time.ids)
X = (dat.in$idtime - mean(dat.in$idtime)) / sd(dat.in$idtime)
# Get spline design matrix for national trend
knots <- quantile(X, seq(0, 1, length=(num.knots+2))[-c(1,(num.knots+2))])
Z_K <- (abs(outer(X, knots,"-")))^3
OMEGA_all <- (abs(outer(knots, knots, "-")))^3
svd.OMEGA_all <- svd(OMEGA_all)
sqrt.OMEGA_all <- t(svd.OMEGA_all$v %*%
(t(svd.OMEGA_all$u)*sqrt(svd.OMEGA_all$d)))
Z <- t(solve(sqrt.OMEGA_all,t(Z_K)))
# Get spline design matrix for area deviations (2 knots only)
knots <- quantile(unique(X), c(1/3, 2/3))
Z_K <- (abs(outer(X, knots,"-")))^3
OMEGA_all <- (abs(outer(knots, knots, "-")))^3
svd.OMEGA_all <- svd(OMEGA_all)
sqrt.OMEGA_all <- t(svd.OMEGA_all$v %*%
(t(svd.OMEGA_all$u)*sqrt(svd.OMEGA_all$d)))
Zarea <- t(solve(sqrt.OMEGA_all,t(Z_K)))
nRiskYears = length(unique(dat.in$RiskInterval))
nage = length(unique(dat.in$bagegrp))
ngroup = length(unique(dat.in$cancergrp))
constants <- list(n = nrow(dat.in),
d.star = dat.in$d_star, y = dat.in$y,
RiskYear = dat.in$RiskInterval,
agegp1 = as.numeric(dat.in$bagegrp == 1),
agegp2 = as.numeric(dat.in$bagegrp == 2),
agegp4 = as.numeric(dat.in$bagegrp == 4),
sex = dat.in$sex,
cancergrp = dat.in$cancergrp,
X = X, Z = Z, Z_area = Z_area, num.knots = num.knots,
areaid = dat.in$idarea,
idtime = dat.in$idtime,
nRiskYears = nRiskYears, nage = nage, ngroup = ngroup,
nAreas = N, scaling.factor = scaling.factor,
adj = adj.info$adj, weights = adj.info$weights, num = adj.info$num, L=L
)
# Initial values
inits <- list(
alpha = rnorm(nRiskYears), # risk interval offsets
beta = rnorm(nage-1), # age group coefficients
gamma = rnorm(1), # linear component of national temporal trend
zeta = rnorm(1), # sex effect
nu = c(0, rnorm(ngroup - 1)), # cancer group effect, cancergrp == 1 is the reference
g = rnorm(num.knots), # spline coefficients for national trend
h = matrix(rnorm(2*N), ncol = 2), # spline coefficients for area deviations
theta = rnorm(N), # unstructured random effect
phi = rnorm(N), # ICAR
taugam = rgamma(1, 0.1, 0.1),
taug = rgamma(1, 0.1, 0.1),
tau.u = rgamma(1, 0.1, 0.1),
tauh = rgamma(1,1,0.1),
rho = rbeta(1,1,1))
# Set initial values for h to sum to zero for all knots, k
for (k in 1:ncol(inits$h)) inits$h[,k] = inits$h[,k] - mean(inits$h[,k])
source(nimble.model.code)
# Parameters to monitor in Nimble
parameters2 <- c(grep("^h$|theta|phi", names(inits), value = T), "d.excess")
parameters <- c(setdiff(names(inits), parameters2))
Rmodel <- nimbleModel(model.code,
data = list(d = dat.in$d),
inits = inits,
constants = constants
)
mcmcConf <- configureMCMC(Rmodel,
monitors = parameters,
monitors2 = parameters2
)
# Place block sampler on national trend parameters
tmp = c(paste0("g[", 1:num.knots, "]"), "gamma")
mcmcConf$removeSamplers(tmp)
mcmcConf$removeSamplers(tmp)
mcmcConf$addSampler(target = tmp,
type = "AF_slice")
Rmcmc <- buildMCMC(mcmcConf, nchains = 1)
Cmodel <- compileNimble(Rmodel)
Cmcmc <- compileNimble(Rmcmc, project = Rmodel)
mcmc.out <- runMCMC(Cmcmc,
niter = 100000,
nburnin = 50000,
thin = 5,
thin2 = 50)
9.4.5.1.5 Nimble code
library(nimble)
model.code <- nimbleCode({
for (idx in 1:n) {
d[idx] ~ dpois(d.excess[idx] + d.star[idx])
log(d.excess[idx]) <-
log(y[idx]) +
alpha[RiskYear[idx]] +
beta[1] * agegp1[idx] + beta[2] * agegp2[idx] + beta[3] * agegp4[idx] +
zeta * sex[idx] +
nu[cancergrp[idx]] +
gamma * X[idtime[idx]] +
g[1] * Z[idtime[idx],1] + g[2] * Z[idtime[idx],2] +
delta[areaid[idx]] +
h[areaid[idx], 1] * Z_area[idtime[idx],1] +
h[areaid[idx], 2] * Z_area[idtime[idx],2]
}
for (al in 1:nRiskYears) { alpha[al] ~ dnorm(0, 0.01) }
for (be in 1:(nage-1)) { beta[be] ~ dnorm(0, 0.01) }
gamma ~ dnorm(0, taugam)
for (k in 1:num.knots) { g[k] ~ dnorm(0, taug) }
zeta ~ dnorm(0, 0.01)
for (gdx in 2:ngroup) { nu[gdx] ~ dnorm(0, 0.01) } # cancergrp == 1 is the reference
for (i in 1:nAreas) {
delta[i] <- (1/sqrt(tau.u))*(sqrt((1-rho))*theta[i] + sqrt(rho/scaling.factor)*phi[i])
theta[i] ~ dnorm(0, tau = 1)
for (kk in 1:2) { h[i,kk] ~ dnorm(0, tau = tauh) }
}
phi[1:nAreas] ~ dcar_normal(adj[1:L], weights[1:L], num[1:nAreas], tau = 1, zero_mean = 1)
rho ~ dbeta(1,1)
# Priors of precision parameters
taugam ~ dgamma(1, 0.01)
tauh ~ dgamma(1, 0.01)
tau.u ~ dgamma(1, 0.01)
taug ~ dgamma(1, 0.01)
})